The unpredictability of life outcomes
Dr. Matthew J. Salganik discussed the impact family background and childhood experiences can have on life outcomes in the first annual MDI Distinguished Lecture.
This fall, the McCourt School’s Massive Data Institute (MDI) launched its Distinguished Lecture Series to bring leading scholars from around the world to Georgetown University to share cutting edge research at the intersection of technology and policy.
The first annual Lecture featured Dr. Matthew J. Salganik, a professor of sociology at Princeton University and author of “Bit by Bit: Social Research in a Digital Age ,” who discussed his research on the unpredictability of life outcomes, specifically the impact of social circumstances on adolescent success.
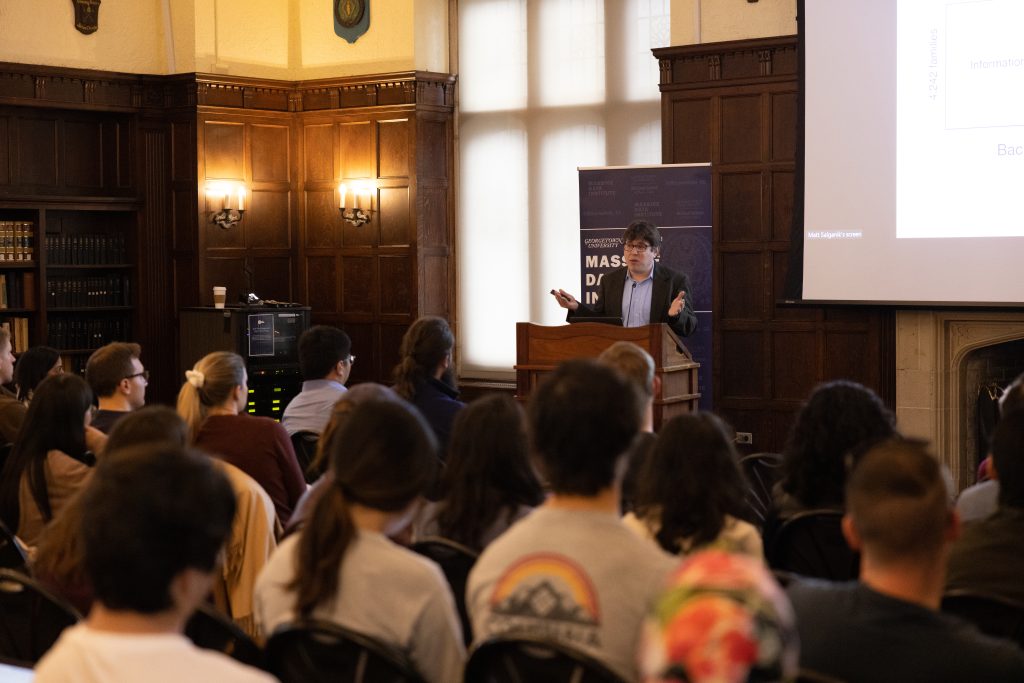
In early 2020, Salganik and his colleagues began their research utilizing data from the Fragile Families Challenge (FFG), a mass collaboration among social and data scientists that combines predictive modeling, causal inference and in-depth interviews to yield insights that can improve the lives of disadvantaged children in the United States. Salganik took a novel approach to determining the predictability of the impact social circumstances have on adolescent success. By combining the theories of public policy experts and data scientists, he developed a machine learning algorithm that utilized prediction in service of understanding societal issues with greater detail than social science could on its own.
The 6 key outcomes used to predict adolescent success
Salganik’s research project centered on about 4,000 families who participated in a multi-year series of surveys disseminated by FFG on the social circumstances of caregivers and children. Salganik and his team analyzed data for six key outcomes for each child: GPA, psychological grit, housing status, material hardship, primary caregiver job training and primary caregiver job loss. He then developed a model to predict GPA outcomes given a student’s particular background or set of circumstances. In the absence of certainty, Salganik took a step back to focus on the “white space” that existed between the model predictions and actual outcomes.
In his latest research, Salganik and his colleagues are on a mission to develop an ideal data pipeline that will be able to train models to predict outcomes with a much higher degree of certainty. Thus far, their model seems to be working and has more credibility and scalability than their previous work.
In the future, Salganik hopes to apply similar models to other societal issues like voter participation and access to health care.